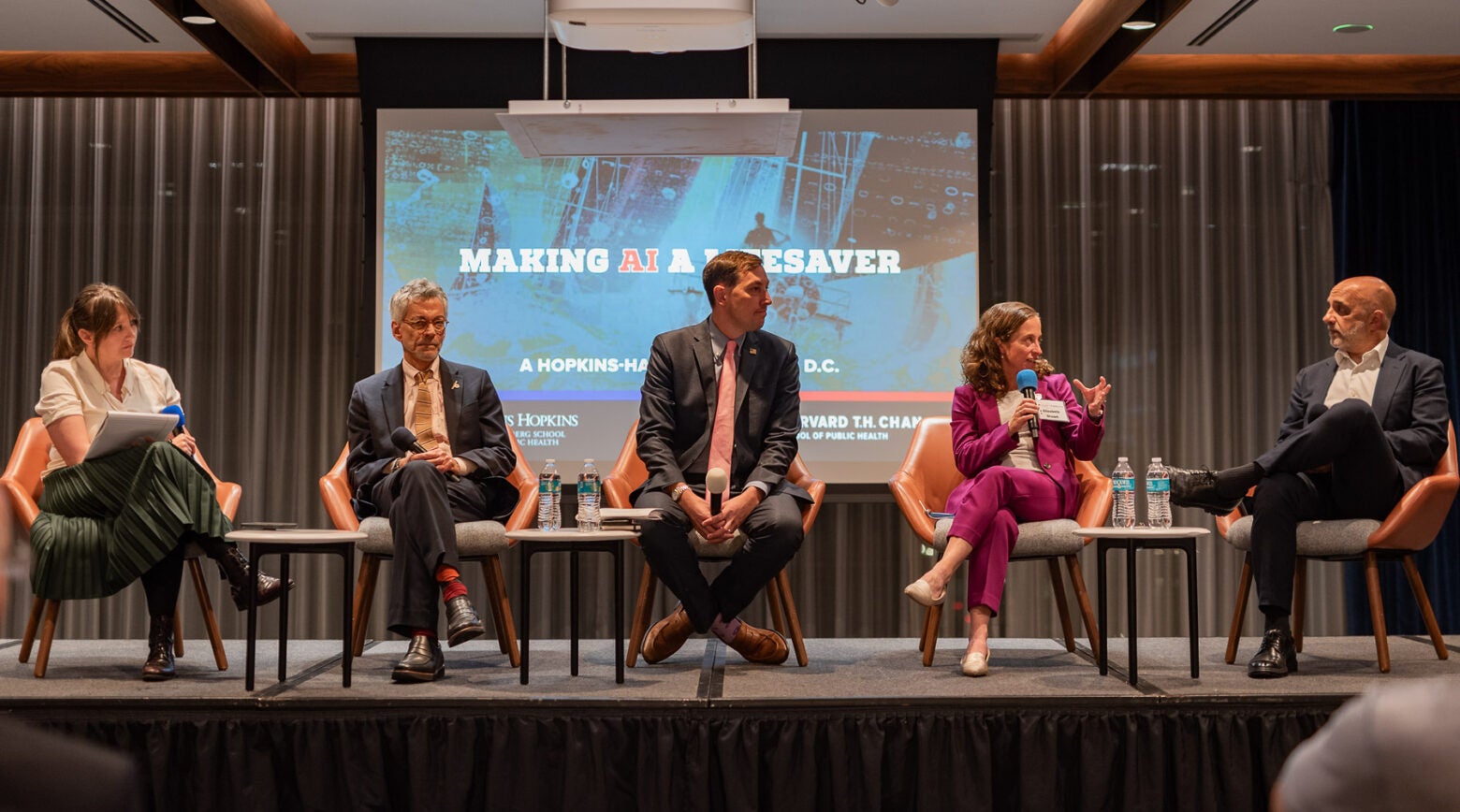
Ideas
The path to more equitable AI
This story was co-published with Global Health NOW. Subscribe to its newsletter.
Public health experts extolled the promise of artificial intelligence to solve longstanding public health problems in a panel discussion on AI but also raised concerns about its potential for exacerbating inequity.
An example of the technology’s potential was how AI was used by the Chicago Department of Public Health to make outbreak predictions for diseases, such as measles. The technology could be applied widely to forecast and prevent food-borne illnesses, said Micky Tripathi, acting chief AI officer at the Department of Health and Human Services. The United States has vast discrepancies in regulatory approaches at different levels of government, as well as in the size and sophistication of local public health staffs. “How do we figure out how these technologies can be democratized?” he asked. Minimizing such gaps is a primary concern for HHS as it prepares a strategic plan for AI.
Tripathi made his remarks as part of the panel “Making AI a Lifesaver,” held on October 8 at the Johns Hopkins University Bloomberg Center in Washington, D.C. The panel was cosponsored by Harvard Public Health, Global Health NOW, and Hopkins Bloomberg Public Health.
Sign up for Harvard Public Health
Delivered to your inbox weekly.
Another panelist, John Auerbach, senior vice president at the global consulting firm ICF, noted that AI could help small public health departments by streamlining tasks like filling out forms or using AI to decide which restaurants to inspect. But he asked, “how do you compensate for the fact that there’s not going to be sophisticated data capacity in a lot of locations?” He said using AI equitably might require a “slow” and “simple” approach oriented more toward everyday tasks than visionary applications.
The panelists delved into AI’s potential to shake up health care to improve both efficiency and outcomes of care. Possible uses range from vaccine and drug development to medical diagnostics and disease screening to providing personalized health messaging to patients. Right now, though, AI is primarily popping up in assisting diagnostics in radiology and in routine administrative applications. While there are myriad examples of AI pilots, things that scale are far less evident.
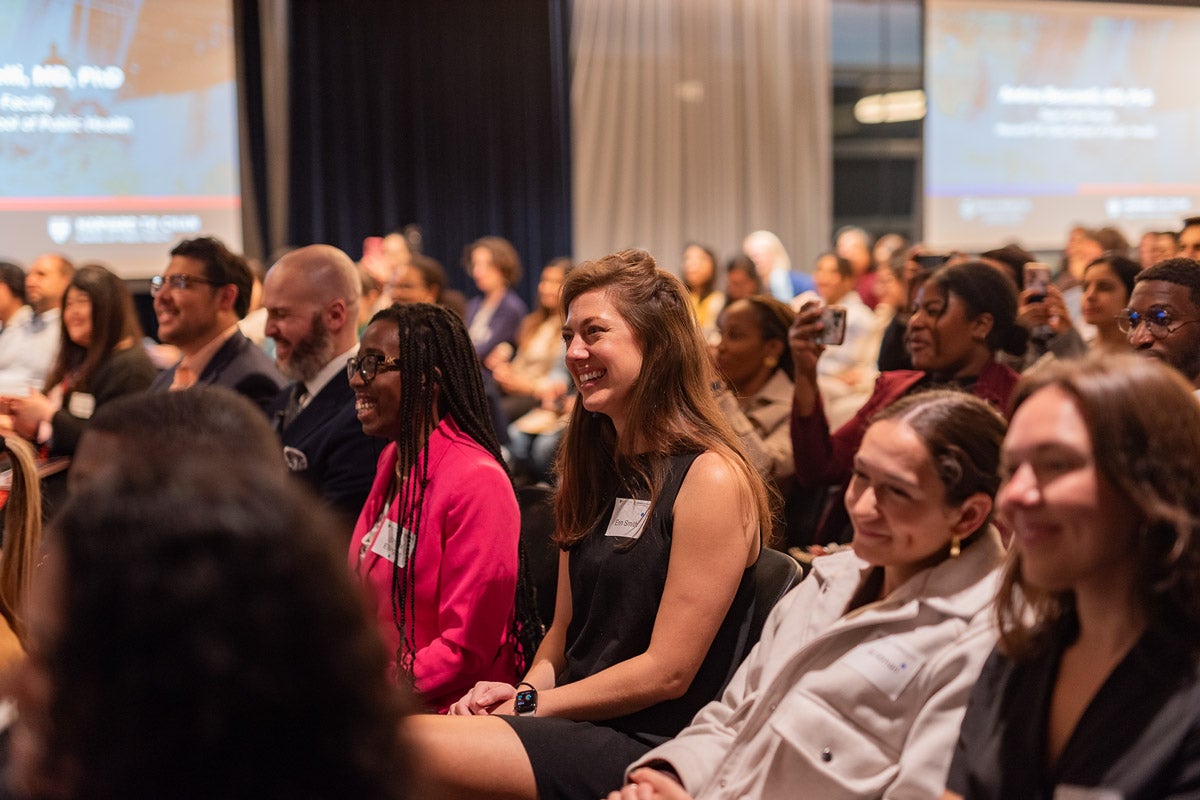
Audience members listen to speakers at an event entitled “Making AI a Lifesaver," held on Oct. 8 at the Johns Hopkins University Bloomberg Center in Washington, D.C.
Disparities in health care resources hamper the equitable use of AI. For one, developing AI applications is costly. A single AI model can cost upwards of $1 million, beyond the reach of under-resourced health departments and hospital systems. One panelist said a dean at Stanford University told him the school had spent between $3 and $5 million on a single AI implementation. “Nobody can scale that for implementation, right?” said Jesse Ehrenfeld, a radiologist and immediate past president of the American Medical Association. Another panelist, Elizabeth Stuart, a biostatistician at the Johns Hopkins Bloomberg School of Public Health, noted that AI continues to draw on limited data sets, a problem in both research on and application of the technology. “We need to be really conscious of who is not in the data that we are using to develop these models, and then the implications of that for use in various settings,” Stuart said.
There’s already a practical divide around AI emerging in public health departments. One survey of local health departments in the U.S. found that among those serving a population of over 500,000, 24 percent were already engaging in AI or had plans to do so, versus only five percent of smaller departments.
Avoiding an AI double standard is possible, the panelists said. One way to expand access is to develop AI platforms that are openly accessible and can seamlessly integrate with different health data sources and software across different care settings.
Several efforts are underway to bridge the AI gap. In January, the National Science Foundation unveiled the National Artificial Intelligence Research Resource pilot, a two-year program aimed at lowering the barriers for innovation in AI. The program connects successful applicants to infrastructure resources for developing new AI models.
Voluntary academic-led collaborations are also accelerating the adoption of AI in health care. Institutions such as the University of California health systems and Duke University are partnering with various health care providers to share AI research, validation practices, and standards for AI use. Tripathi said public-private partnerships in AI are essential, and because of the U.S.’s federalist nature, AI policy related to public health is certain to vary by state.
The panelists broadly agreed that there needs to be more transparency in how AI is being used. For starters, noted Ehrenfeld, better visibility into AI will help flag flaws that lead to inequity as well as make AI a more effective tool for public health workers. Stuart noted the clear need for training on AI’s ethical issues and applications presents a big opportunity for schools of public health and medical schools.
To counter AI’s transparency challenges, policymakers are working to improve the regulatory structures. Last October, the Biden administration signed an executive order to accelerate the ethical management of AI’s risks. It tasked HHS with drafting an AI action plan to oversee responsible AI implementation in healthcare.
Tripathi said strategies include a certification system for companies that sell electronic health records. To gain this imprimatur, vendors who build an AI application need to disclose the model’s training data set, maintenance strategies, and validation methods. The published information is “basically a nutrition label,” he said. If every vendor in the U.S. gained certification, it would cover 96 percent of hospitals and 78 percent of physician offices nationwide.
Tripathi noted that HHS plans to release its full strategy for AI in January.
One thing that appears unlikely is Congressional action to help standardize AI policy. “Congress doesn’t appear to be on the verge of having some national stance for any of the states,” Tripathi said. “The notion of states’ rights is something that, if anything, is becoming even more ingrained in this kind of policy.”